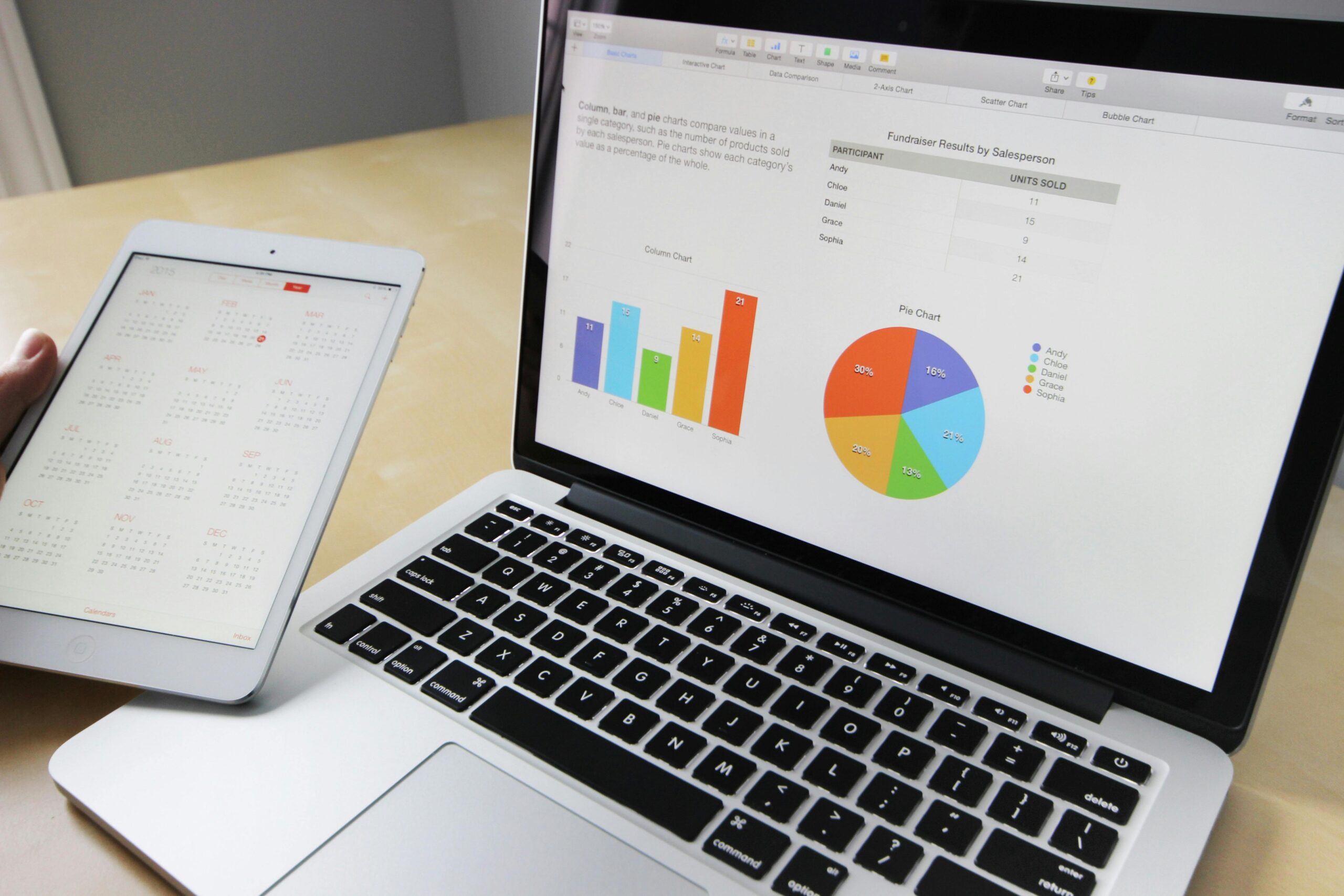
5 Key Metrics for Monitoring Data Quality in 2025
by
For many brands today, customer identity data keeps the gears in motion. This kind of data empowers you to provide a premium, heavily personalized experience for each customer, even on a large scale.
The problem? With countless solutions across your enterprise and data flowing in from every direction, this information can quickly become disorganized, fragmented, redundant, or otherwise compromised—and the customer experience suffers as a result.
Inaccurate, invalid, and disconnected consumer data can leave its mark on your business, causing issues and frustrations that can bog down call centers, hinder the customer experience, increase compliance risks, and leave ROI on the table. That’s why it’s so important to monitor all this data to maintain its quality.
Which data quality metrics should you be tracking—and how do these affect the decisions your team makes every day? Here’s a look at why data quality matters, and which data quality metrics deserve your attention this year.
Why Does Data Quality Matter?
Today, data is a precious commodity—with some even equating its immense value to the “new oil.” However, just like crude oil and fossil fuels freshly extracted from below the earth’s surface, data is only useful and valuable when it has been refined.
Data with lots of inaccuracies or other issues can cause you to make the wrong decisions for your business, which can lead to all kinds of problems:
- Insufficient or ineffective marketing campaigns
- Problems or inaccuracies with inventory levels
- Costly decisions regarding the future of your business
- And other costly, frustrating issues that can impact your operations
How do you address these challenges? By prioritizing data quality. There’s a lot that organizations can do with data today—and with high-quality data, you can have more faith in your business insights, make more accurate predictions about how to prepare for the future, and create more personalized experiences for clients and customers.
Low-quality data can have a hugely negative impact on your business. It’s estimated that poor data quality costs organizations an average of $12.9 million every year, often as a result of wrongly-informed decision making.
As the adoption of AI-driven solutions increases exponentially, so too will the need for high-quality data. After all, the bedrock of these AI- and machine learning-powered tools is data; but this data must be clean. In other words, you need high-quality data that’s free of duplicates, that works together enterprise-wide, and that’s not riddled with inaccurate, incomplete, outdated, or incorrectly formatted data. High-quality data can make all the difference in how well these tools support your operations, decision-making, and customer experience.
With these AI-powered solutions, data quality is directly correlated to the customer experience. High-quality data can make all the difference in your customer insights, empowering you to provide personalized experiences, engage with customers, and gain a deeper understanding of your target audience for both marketing strategies and customer communications.
Here’s a look at the factors you should be monitoring in your data quality metrics:
Data Quality Metric #1: Accuracy
How accurately does your data describe whatever is being described? Data accuracy tracks just how correctly your entities or attributes are reflected.
You can also think of data accuracy as how closely your data matches reality. Why is it important to track data accuracy as a metric? Because when data enters your enterprise—either when it’s manually entered or it enters through your IT systems, it might have errors. These anomalies can impact your analytics or even the outcomes of predictive analytics solutions.
Accuracy can also include the uniqueness of your data. In other words, are there duplicates, overlaps, or redundancies?
For example, believing you have two customers or guests when in actuality, a customer’s data is flowing into two separate profiles can create issues for your team down the road—including customer-facing issues that affect the customer experience.
Data Quality Metric #2: Completeness
Is missing data holding you back from getting a true representation of where things are—and where things are heading? Data completeness examines just how much data is missing from any given dataset.
The completeness of your data matters in the same way that having all the pieces of a puzzle matters; without all the data, you don’t get the full picture.
Data completeness evaluates whether all the necessary and relevant data is available. If only some of the data is available, some analytics models may not work, or may not give you wholly accurate results. Incomplete data may be easier to catch than inaccurate data.
Data Quality Metric #3: Consistency
It’s estimated that for every enterprise, each department uses between 40 and 60 different applications—and enterprise-wide, some organizations use as many as 200 applications. While your organization may not use hundreds of applications—and while they may not all need to share the same datasets—there’s still a lot of data that needs to flow freely between solutions. However, if this data doesn’t sync up, you may end up with inconsistencies.
Data consistency takes a look at how many—or how few—contradictions exist within your data.
If there is inconsistent information across datasets, you may struggle to stay updated. For example, if customer data needs to flow between departments, you may find it difficult to know what information is the most current or accurate for each customer.
Data Quality Metric #4: Timeliness
Is the data your organization working with updated in real-time—across the entire enterprise? What’s more, is it available to your teams when they need it?
Delays in sharing this data can impact analytics, and even the customer experience. Data timeliness refers to how current and available your data is organization-wide, especially concerning how you intend to use this data.
Customer experience data, in particular, requires up-to-date data to be successful. CX data needs to respond in real time so your entire organization can respond to each customer’s needs and attend to their preferences for a truly personalized experience at scale—and one that’s seamless from one channel or touchpoint to the next.
Data timeliness as part of the customer experience can also help support issue resolution. Both issue resolution and personalization are essential to establishing customer satisfaction and building customer loyalty. Timeliness can also help you react to customer behaviors and market trends as they evolve.
Data Quality Metric #5: Validity
Is your data valid; in other words, does it follow your organization’s necessary formats and constraints? Data validity can affect how accurate and complete your entire dataset is, as well as how each piece of data fits with your established rule sets and ranges.
Identity data validity may include things like:
- Invalid dates
- Incorrectly entered phone numbers
- Wrong email addresses
- Misspelled customer names
- And more
Data validity can have a huge impact on the customer experience—especially as this data flows between solutions. Not only can this negatively affect the customer experience, but it may create issues with data accuracy and relevance for your internal teams as well.
How harpin AI Supports High-Quality Data
At harpin AI, we understand that the quality of your data is directly linked to your performance. With a team of experts on staff and advanced AI/ML technology, we offer real-time data pre-processing to level up your data quality and transform your IT operations, sales, marketing, call centers, compliance, and AI readiness teams.
What does this mean for you? More accurate, accessible, and actionable entity data, which translates into more efficient operations and a more personalized customer experience from Day 1.
What’s more, we seamlessly integrate with your existing systems, supercharging your investments instead of replacing them, for cohesive, accurate, and compliant entity data at the highest level.
It means you get a clear, holistic understanding of your customers for more targeted marketing, more customized interactions with individual customers, and stronger predictive analytics—based on accurate, high-quality data you can trust.
Discover what you can do with high-quality data through harpin AI. Book a demo today!